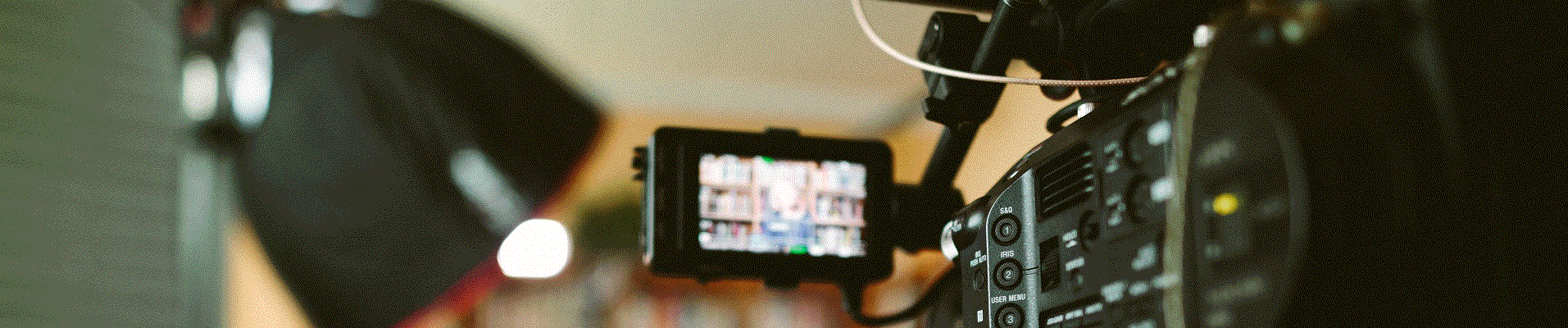
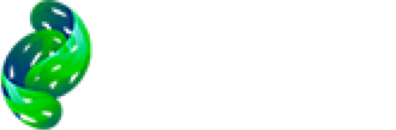
"Can machines think?" In 1950, Turing raised an epoch-making question that makes sense in the fields of computer science and AI till today.
Turing might haven't expected when he raised this question that AlphaGo, an AI robot shocked the world by beating the world Go champion in 2016, and that an AI tool named AlphaFold2 unveiled the reform of life science after it successfully predicted 98.5% of the structure of human proteins in 2021. We're embracing a brand new era of digital civilization.
A new era, however, comes along with new challenges. Computation speed is subject to Von Neumann architecture, computational method is challenged by mega data, the supply of computational power is up to energy consumption, the use of computation is restricted by means of access... We haven't thoroughly tapped the intelligence and computing potentials and see too many unknowns ahead of us waiting to be solved.
ZJ Lab, together with Science, joins hands with experts and scholars from all around the world to put forward 10 fundamental scientific questions of great significance to future intelligent computing research, which were officially released at the 2nd Innovation Forum on Intelligent Computing unveiled today.
We hope that like the far-reaching questions raised by our great forerunners, the 10 fundamental scientific questions on intelligent computing will provide guidance for intelligent computing research and inspire us to explore the knowledge boundary of intelligent computing and further tap the potential of intelligent computing. We look forward to working with all scientists to make breakthroughs and technological progress, so as to pave way for our journey to digital civilization and enable the wide application of intelligent computing in scientific research, industrial development and social governance.
10 Fundamental Scientific Questions on Intelligent Computing
1. How do we define intelligence and establish the evaluation and standardization framework for intelligent computing?
Broadly speaking, intelligence is the ability to analyze and appropriately respond to input (data). Many say that a truly intelligent system should be able to adapt to its environment—to learn, to reason, and to evolve. Yet how can we know whether that is the case for any given system?
The traditional evaluation of whether a system is intelligent is the Turing Test—can a human distinguish whether the system is a human or computer? Other, weaker, metrics exist such as asking whether the system performs its designated tasks accurately, or whether it can generalize beyond the data it has been trained on. The rules for evaluation should be dependent on broader social contexts that allow for fairness and transparency.
Whether a standard framework for intelligent computing can be established is still an open question, as there is no universally agreed-upon metric upon which to conduct the debate. The rules pertinent to one system may run afoul of rules established for another, and the sands upon which that system is built may shift.
2. Is there a unified theory for analog computing?
Analog computing uses hardware to simulate algorithms, measuring continuous signals such as voltage or light intensity. It offers the advantages of low energy consumption and high computing efficiency in solving specific problems. But it fell out of favor many years ago with the advent of digital computing (which counts instead of measures), in part because at that time it was difficult to scale up and to verify analog systems.
Yet because of its ability to mimic components of biological networks such as synapses and neurons, analog computing has seen a resurgence. Different algorithms and platforms have evolved, all trying to establish more efficient ways to measure in the analog domain.
At present, though, it is an unrefined practice, using many kinds of physical carriers and calculation methods for simulation and calculation. It awaits a unified theoretical model to help promote its standardization and large-scale application.
3. Where will the major innovations in computing come from, and will quantum computing approach the computational power of the human brain?
Joint design and coevolution of hardware and software will likely be a driving force behind major computing advances. Innovation is coming from all levels: We’re seeing breakthroughs in emerging devices with unique properties almost every year. These drive—and are driven by—how they are organized into circuits and hierarchical systems, then into the algorithms and applications in which they are deployed.
Some new devices may not be useful for conventional computing, but might make neural networks efficient, while newer computing models may need unconventional hardware support. For example, new architecture will be needed to emulate the behavior of astrocytes (star-shaped glial cells in the central nervous system), which have been found to play an important role in cognition and differ in significant ways from neurons.
Quantum computers are operated differently from general purpose computers. It is still early in their development—currently they are mostly used for massive number-crunching activities such as encryption. Whether they will someday be able to simulate the cognitive-computing and even emotive ability of the human brain is a matter of active research.
4. What new devices will be built (transistors, chip design, and hardware paradigms: photonics, spintronics, biomolecules, carbon nanotubes)?
These and other devices already exist, or are actively being researched, at the nanometer scale, and further scaling is likely. The key is to make them better and make better use of them.
For example, there are many devices that are essential resistors, which can be programmed into levels, and those levels are memorized and transferred. A variety of technologies—electronic, photonic, etc.—can exhibit very similar behavior. These may be made to act very much like synapses in the brain in that the signal can be transferred, amplified, or reduced, and the excitations are integrated to build up synaptical waves that will be the basis of universal devices.
An issue is how to combine multiple physical dimensions, such as wavelength and polarization modes, to develop the corresponding optoelectronic interconnection devices. Power, performance, area, and cost need to be addressed to scale the technologies and allow them to evolve.
5. How could intelligent computing enable intelligent machines?
The term "machine" is an essential concept for "computing." A machine—intelligent or otherwise—primarily has three components: a sensor that gathers external excitations (data), a memory that stores the information collected by the sensor, and a logic unit that collects data from the memory and performs inferences upon it, taking actions or sending signals.
An intelligent machine will perform intelligent computing. The question then becomes whether we can create an intelligent computing paradigm.
6. How can we understand the storage and retrieval of memory based on the digital twin brain?
The spatiotemporal dynamics of memory storage and retrieval suggests that it is highly controllable, giving hope that faulty memory can be repaired. Yet the synergistic and dynamic nature of brain networks hinders the exploration of the complex properties of memory.
Researchers have already created digital twins of different organs, including the brain, modeling and simulating their multiscale structure and function for research into pathologies such as Alzheimer's disease and epilepsy. While these simulations are arguably much less complex than human memory, they do demonstrate a proof-of-concept. Digital twins of the brain and its parts should allow researchers to break through the spatiotemporal scale and accuracy limitations of existing research into memory, its pathology, and its modulation.
Memory comprises the connections between the senses, emotions, concepts, and motor movements. As such, even if we succeed in replicating the entire brain, we cannot ignore those connections.
7. What is the most efficient path to converge silicon-based and carbon-based learning?
Silicon-based computing is gradually reaching its physical limits. Meanwhile, the human brain—the highest known form of carbon-based computing—lacks the speed, accuracy, and reliability of silicon. Carbon- and silicon-based computing platforms differ from each other in myriad ways. The former relies on a sparse but highly connected network of neurons, which is slow in terms of signal processing but very good at certain applications. Silicon platforms, on the other hand, rely on a highly integrated two-dimensional layout that boasts much faster transfer speeds.
Researchers are investigating at least two pathways to converge these systems: One is to build a mathematical model of the neural network based on current silicon-based architecture. Another is to build deep neural networks with layers upon layers of network connections.
In their current incarnations, simple interconnects don't do computing. Perhaps one path to convergence would include building components that act more like neuronal synapses, integrating information and participating in the computational processes, rather than just acting as a relay.
8. How to build interpretable and efficient AI algorithms?
Efficient artificial intelligence (AI) algorithms with interpretability have long been pursued. Can new mathematical methodologies such as tensor networks, combined with the effective integration of expert knowledge, logical reasoning, and autonomous learning, bridge the divide between interpretability and efficiency in AI technology? Will that integration break the existing status of deep learning as "black box algorithm" and establish a new generation of interpretable systems that can be applied to different fields and different scenarios (voice, image, video, digital twin, metaverse, etc.)?
9. Can strong intelligent computing with features of self-learning, evolvability, and self-reflection be realized?
The goal of intelligent computing is to solve large-scale complex problems efficiently and autonomously in the human–machine–object space. The approaches of weak intelligent computing (weak AI) can obtain good results for such problems to a certain extent, but essentially, they rely heavily on the customized input of human a priori knowledge such as artificially preset physical symbol systems, neural network models, and behavioral rule sets.
Strong intelligent computing (strong AI) can change dynamically depending on the input and the environment. In different contexts, self-learning ability allows the system to avoid repeating the output of previous internal states; evolvability allows the system to adaptively improve its architectural pattern; self-reflection enables the system to expand the generalizability of the model based on the experience of solving historical tasks. Therefore, one of the fundamental scientific challenges for future intelligent computing is to study the computing theory of higher-order complexity and to explore the automatic construction paradigm for solving major scientific problems, as well as letting the computer independently perform task comprehension and decomposition, optimized dynamic path construction, and kernel development model and evolution.
10. How can we use real-world data to discover and generalize knowledge?
There is a significant argument in the computing field as to whether machine learning can truly generalize, or whether it simply reiterates what is already known in a more efficient manner. Being able to identify objects or labels in a test set, it could be argued, is nothing more than saying that this object shares sufficient characteristics with those that were used to define it in the first place.
Therefore, intelligent computing needs to complete the calculation tasks originally performed by human predefined logic in an active, heuristic, and open intelligent form, and the effectiveness of these calculations need to be verified in the real world. Knowledge discovery is the premise of knowledge-driven applications, which makes it a significant indicator of how strong AI is. Knowledge discovery of real world data is a major scientific problem to be solved by intelligent computing.The ability to be active and heuristic in open-world computing is an important milestone for intelligent computing to reach if it is to perceive anomalies, discover rules, summarize knowledge, and solve the limitations of logic program execution through finite-state machines.
The above is a compilation of contributions from (in alphabetical order):
CHEN Yiran (Duke University)
FENG Dawei (China Computer Federation)
JACOB Ajey (University of Southern California)
JIANG Tianzi (Chinese Academy of Sciences)
JIN Shangzhong (Zhejiang Lab)
LI Zhao (Zhejiang Lab)
LI Deyi (Chinese Association for Artificial Intelligence)
LIU Wei (Beijing University of Posts and Telecommunications)
MENG Lei (Shandong University)
QIU Jiong (Zhejiang Zelian Technology Co.)
QIU Qinru (Syracuse University)
RAN Shiju (Capital Normal University)
SHANG Hongcai (Beijing University of Chinese Medicine)
SHI Yiyu (University of Notre Dame)
SHI Tuo (Zhejiang Lab)
SU Gang (University of Chinese Academy of Sciences)
WANG Huaimin (China Computer Federation)
WANG Tao (China Computer Federation)
WANG Zhiwei (AGI-Lab Shanghai)
XIONG Chuyu (Chengdu Cyberkey Technology Co.)
XU Kele (China Computer Federation)
YAN Junchi (Shanghai Jiao Tong University)
YU Fei (Carleton University)
ZHAO Zhifeng (Zhejiang Lab)
ZHANG Ji (Zhejiang Lab)
ZHANG Yu (Zhejiang Lab)
ZHOU Tianshu (Zhejiang Lab)
ZHU Shiqiang (Zhejiang Lab)